Bristol University celebrates three years of EPIC-KITCHENS
Computer vision could revolutionise all aspects of everyday life. From shopping to medical care, to transportation, there’s a high chance that many of these future applications will be possible thanks to ground-breaking research at Bristol.
Celebrating its third anniversary this month, the EPIC-KITCHENS project marked a pivotal moment in the field of computer vision as it became the world’s largest unscripted first-person (egocentric) vision research dataset, opening doors to all kinds of real-world applications for the very first time.
After nearly 5,000 downloads from 42 countries, and mentions in more than 400 academic papers, the project – which captured and processed the everyday kitchen activities of people in their own homes – has also caught the attention of the Defense Advanced Research Projects Agency (DARPA). The world-famous research and development facility is now inviting proposals based exclusively on the project’s research.
In short, it’s a pioneering project that’s going to have, and is already having, a significant impact on the evolution of computer vision technology.
Understanding computer vision
Computer vision is a subfield of artificial intelligence. Its purpose is to program a computer to ‘understand’ an image, or features of an image (either static or moving), with lots of different goals in mind – for example, recognising particular objects or actions, or how elements of an image typically correspond to one another.
Once the computer is able to understand what it sees, researchers are able to create relevant algorithms for the program, depending on its purpose. Computer vision is a central component of self-driving cars, for example. Once the program has learnt to identify key objects (other vehicles, trees, pedestrians, and so on) researchers are able to create an algorithm that ensures the vehicle avoids these objects through braking or steering.
Computer vision programs require datasets to ‘learn’. Traditionally, these datasets are scripted (that is, an image or video clip has been designed and created specifically for computer vision learning), and they focus on a specific query, such as action recognition (what is the person doing?) or object recognition (what is the object and where is it?).
This is where EPIC-KITCHENS’ research signalled a breakthrough in computer vision data collection: it was entirely unscripted. Instead of recording people ‘performing’ a kitchen-based action for cameras to capture, the study participants wore head-mounted cameras that captured every single thing they did in their own kitchens of their own volition – mistakes, mess, spills and all. Instead of a controlled, precise library of images, the EPIC-KITCHENS dataset involved more than 100 hours of HD recordings, 20 million image frames, 90,000 different actions, and 20,000 unique narrations across multiple languages.
“Other datasets of a similar scale tend to be scripted so the actions don’t look natural, or they contain lots of short clips of video where the goal is to understand the action happening in that particular clip,” explains Hazel Doughty, one of the researchers on the EPIC-KITCHENS team. “But events don’t happen in isolation, so the ability to look at what someone is doing before and after an action is key to computer vision being able to automatically understand videos.”
No-one else had ever attempted to capture such a large, unwieldy dataset before. “We knew there was a need for this, and we didn’t want to avoid attempting it simply because it was hard,” says Dima Damen, Associate Professor in Computer Vision and the project’s principal investigator. “The fact is, computer vision technology can’t continue to progress with the usual acted set-ups. The longer we spend with scripted datasets, the further we’re getting from our goal of creating AI that is genuinely useful. EPIC-KITCHENS changes that.”
The project in action
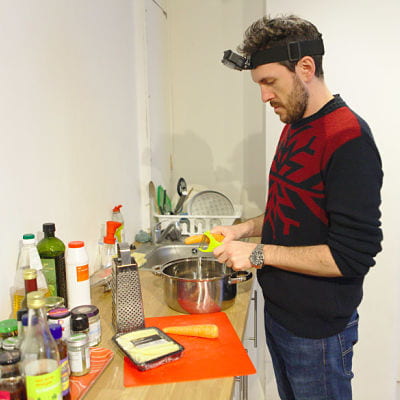
The project kicked off in 2018, with – thanks to several travelling researchers at the time – support from the University of Catania and the University of Toronto. This, Dima says, helped to bring some diversity to the data collected.
There were an initial 32 participants involved, each tasked with wearing a head-mounted GoPro to capture every hand-based action undertaken in their kitchen. Across three days, participants were asked to record everything, from making coffee and washing up, to chopping vegetables and emptying bins.
“We wanted to see the clutter of the typical home environment,” says Dima. “And to observe the actions of participants in an environment they know, where they could move smoothly and efficiently and do multiple things at once. And – importantly – to make natural mistakes. This sort of data capture wouldn’t be possible in a controlled, scripted setting.”
Once the video had been captured, each participant was asked to watch it back and use the project’s novel ‘Pause-and-Talk’ interface to create an annotation of the action they were performing on screen: for example ‘I chop the carrot’ or ‘I am opening the cupboard’. This allowed the research team to assign each action a label, which forms the foundations of a dataset. “By getting the participants to watch their videos back and narrate what they were doing over the top of the video, we were able to obtain rough annotations for what was happening in the video very quickly,” explains Hazel. “These were then refined to be more precise and reflect exactly when these actions started and ended in a fraction of the time it would have taken otherwise.” This, she says, helped to mitigate one of the challenges associated with managing a dataset of this size.
Tessellating saucepans and onion oddities
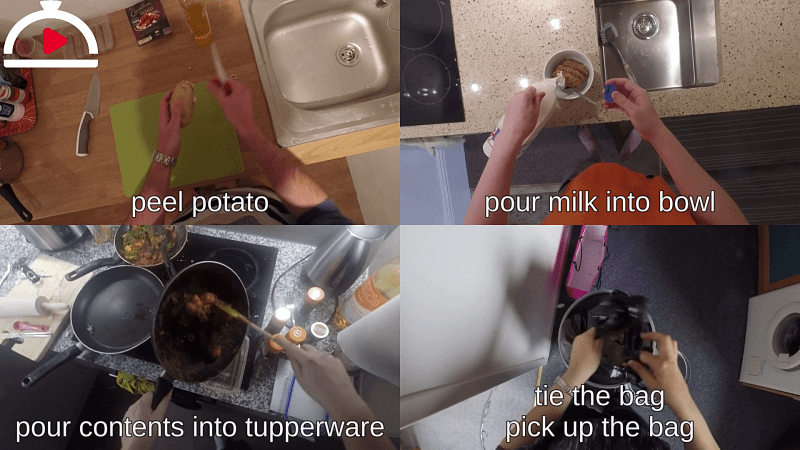
The size and the scope of the data collection was in itself a difficulty, but the team came up against a number of other challenges during the course of the project. Participants whose native language wasn’t English, for example, sometimes struggled to recall the English names of kitchen tools or ingredients during their narrations. “Computers are very good at telling you what objects are in an image, but they’re still a long way off understanding language to the level of a human,” says Michael Wray, a postdoctoral researcher on the team. “Because of this, a lot of time was spent simplifying and translating these narrations to what standard methods are used to. One participant used the phrase ‘tessellate the saucepans’ instead of ‘stacking the saucepans, for example!”
Other challenges were more expected, such as the ‘long tail’ of some distributions of actions. “This means there were lots more examples of one type of action, such as washing a dish, than there were of others, such as slicing an avocado,” says Dima. “Most machine learning datasets have an equal number of every class, so the computer can ‘equally’ learn these examples. In our organic, unscripted kitchens, though, this was impossible.” To mitigate this, the team leveraged a process called disentanglement, where the action was separated from the object. Instead of learning what ‘cutting a cucumber’ looks like, the computer learns what the action of cutting looks like, and separately, what a cucumber looks like, and can then mix and match combinations with other actions and objects.
The research produced some more unexpected outcomes, too. “One thing we hadn’t considered was the role that sound would play in the recordings,” says Dima. “The equipment we used was great quality but when you break it down into individual frames it’s not always easy to tell whether a tap is running, or whether someone is chopping a potato or onion. The audio helped us with that.”
And on the subject of onions, the organic mistakes-and-all nature of the actions captured really helped to drive home the potential applications for this level of computer vision learning. “All of the researchers recorded their kitchen activities as well,” says Dima. “And one day, Hazel came into my office and said, ‘I hope you don’t mind me saying, but I’ve watched some of your footage and you really don’t know how to chop an onion’. It turns out I’d been chopping onions in a really inefficient way all my life and I just didn’t know it! This is a great example of how AI can be genuinely useful in the home.”
But despite the name, EPIC-KITCHENS is not really about kitchens. “I think one thing which may not be obvious to people outside the computer vision research community is that EPIC-KITCHENS isn’t just a dataset for understanding kitchen actions,” says Hazel. “It allows researchers to develop better models for understanding video in general.” As Will Price, PhD researcher on the team, explains, these findings could translate into a wide range of applications for assistive technologies, hence DARPA’s interest. “Imagine a scenario where you are building something or doing a maintenance task and are equipped with a pair of smart glasses. The glasses would be aware of what your task is and your progress throughout the task and would be able to guide and assist you when you make mistakes or complete a step.”
Looking ahead
Three years on, and the EPIC-KITCHENS research has formed the basis of numerous other projects around the world. The original dataset has been expanded, and the project itself lives on through ‘EPIC Challenges’, which encourage researchers to play with the dataset to come up with new solutions to the puzzles still posed by computer vision. The next set of challenge winners will be announced soon.
“There’s a lot of promise in AI and computer vision,” says Dima. “This project is pioneering in that it’s measurably helped to narrow the gap between where the world is with the technology now, and where we want to get to. Thanks to the impassioned efforts and enthusiasm of our dedicated team here at Bristol, we’re one step closer to the future everyone keeps talking about.”
More information
- For information on the dataset and how to participate in its ongoing challenges: http://epic-kitchens.github.io/
- To get in touch, for sponsorship or collaboration, email: uob-epic-kitchens@bristol.ac.uk
- For more about the group’s research: https://dimadamen.github.io